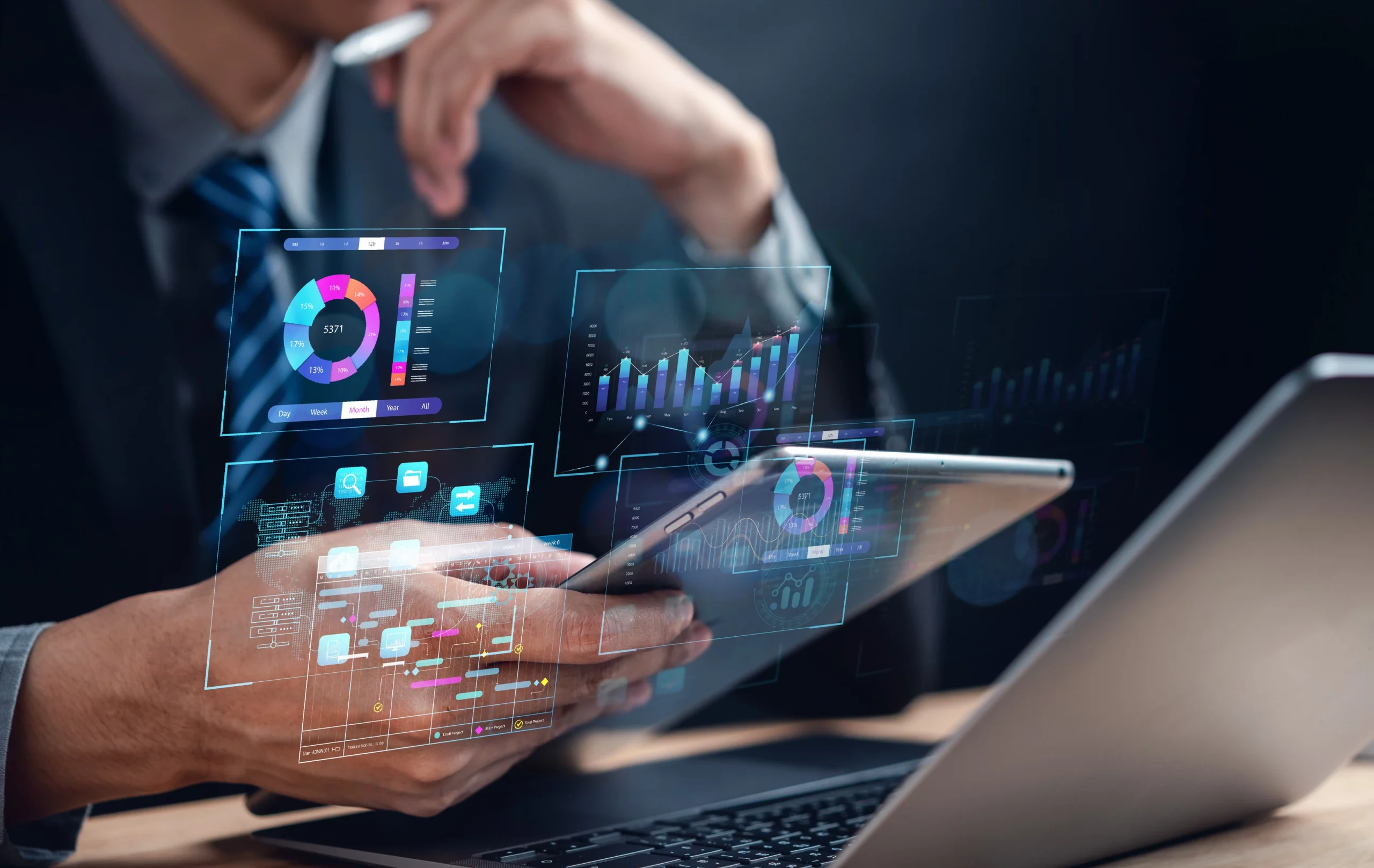
Digital Transformation Journey with DataJob
A recognized Swedish auction company embarked on a digital transformation journey in partnership with DataJob.
Our client, a Stockholm-based company, wanted to transform its business operations using advanced data analytics. The company aimed to improve customer satisfaction and drive profitability by leveraging machine learning (ML).
Machine learning, a type of artificial intelligence, enables software applications to predict outcomes and make decisions without explicit programming. By providing their systems with large amounts of data, our client had the opportunity to identify patterns and characteristics. Over time, the system enabled them to make recommendations, decisions, or predictions based on its experience with the data it processed.
This case study explores how our client integrated machine learning into their operations to enhance business performance, improve customer satisfaction, and drive profitability. It outlines the ML process, highlights diverse applications, and showcases the significant benefits achieved by leveraging ML technologies.
Our client faced several key challenges:
To overcome these obstacles, they required a solution that offered sophisticated data analysis, automation, and predictive capabilities.
The solution we offer to our client is suitable for any business looking to leverage machine learning to enhance operations, improve customer satisfaction, and increase profitability.
This is a general outline of our process:
When implementing machine learning, there are important considerations to keep in mind to ensure its successful integration and operation.
For this project, we ensured a smooth and effective ML implementation aligned with our client’s goals:
Machine learning allows machines to handle complex tasks that would typically require human intelligence, learning from data rather than following a strict and static program code.
Machine learning has diverse applications across industries, including:
Machine learning offers numerous advantages that can drive growth, improve efficiency, and create a competitive edge across various business functions. Some of the key benefits include:
By leveraging machine learning, businesses across various sectors can streamline their operations, enhance customer experiences, and adapt more quickly to changing market demands. This leads to improved efficiency, increased profitability, and a competitive edge in their respective markets.
To learn more about how machine learning can benefit your business, download the case study by filling out the form below or contact us for a personalized consultation and discover the potential of data-driven decision-making.
A recognized Swedish auction company embarked on a digital transformation journey in partnership with DataJob.
DataJob is your Software Consultancy Partner with more than 10 years of local presence in the Nordics.
Cookie | Duration | Description |
---|---|---|
cookielawinfo-checkbox-analytics | 11 months | This cookie is set by GDPR Cookie Consent plugin. The cookie is used to store the user consent for the cookies in the category "Analytics". |
cookielawinfo-checkbox-functional | 11 months | The cookie is set by GDPR cookie consent to record the user consent for the cookies in the category "Functional". |
cookielawinfo-checkbox-necessary | 11 months | This cookie is set by GDPR Cookie Consent plugin. The cookies is used to store the user consent for the cookies in the category "Necessary". |
cookielawinfo-checkbox-others | 11 months | This cookie is set by GDPR Cookie Consent plugin. The cookie is used to store the user consent for the cookies in the category "Other. |
cookielawinfo-checkbox-performance | 11 months | This cookie is set by GDPR Cookie Consent plugin. The cookie is used to store the user consent for the cookies in the category "Performance". |
viewed_cookie_policy | 11 months | The cookie is set by the GDPR Cookie Consent plugin and is used to store whether or not user has consented to the use of cookies. It does not store any personal data. |